Artificial Intelligence (AI) algorithms have the potential to revolutionize education by personalizing learning experiences and optimizing educational outcomes. However, these disadvantages of ai in daily life they are trained on, potentially perpetuating inequalities in educational settings. Mitigating biases in AI algorithms is crucial to ensure fair and equitable learning opportunities for all students.
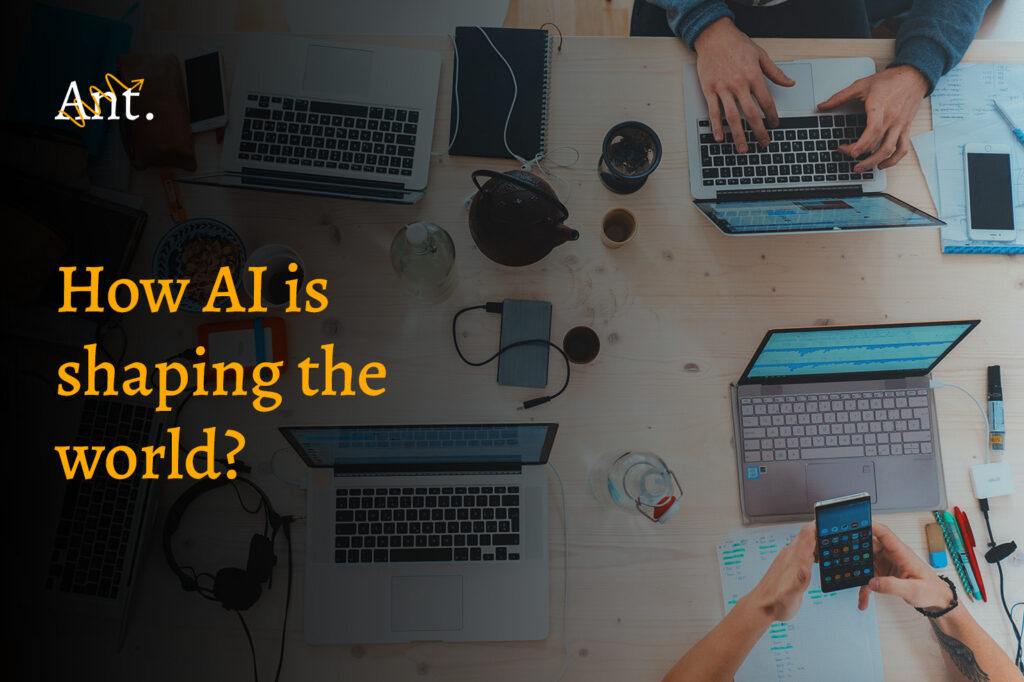
Page Contents
Understanding Biases in AI Algorithms
1. Data Bias
AI algorithms learn from historical data, and if this data reflects societal biases, the algorithms may perpetuate these biases. For example, biased historical grading data could impact algorithmic recommendations, disadvantaging certain groups of students.
2. Algorithmic Bias
Algorithmic biases can arise due to the design or optimization of AI models. Biases might emerge from how variables are weighted or features are selected, leading to unfair decisions or recommendations in educational contexts.
3. Amplification of Inequalities
Biases in AI algorithms can exacerbate existing inequalities in education, impacting access to resources, opportunities, and learning experiences for marginalized or underrepresented groups.
Strategies to Mitigate Bias in AI Algorithms
1. Diverse and Representative Data
Using diverse and representative disadvantages of ai in daily life biases in AI algorithms. Ensuring that training data reflect the diversity of students, considering various demographics, backgrounds, and experiences, can lead to more inclusive algorithms.
2. Continuous Monitoring and Evaluation
Regularly monitoring AI algorithms for biases and evaluating their outcomes is crucial. Implementing ongoing assessments helps identify and rectify biases that may arise during algorithm deployment.
3. Ethical Guidelines and Standards
Establishing clear ethical guidelines and standards for AI development in education is essential. Educators and developers must prioritize fairness, transparency, and accountability in AI algorithm design and deployment.
4. Incorporating Fairness Metrics
Integrating fairness metrics into AI algorithms enables developers to measure and quantify biases. Techniques like fairness-aware machine learning help optimize algorithms to minimize disparities in outcomes.
5. Collaborative Approach and Diverse Perspectives
Involving diverse stakeholders, including educators, students, ethicists, and community representatives, fosters a collaborative approach to address biases in AI algorithms. Diverse perspectives contribute to more comprehensive solutions.
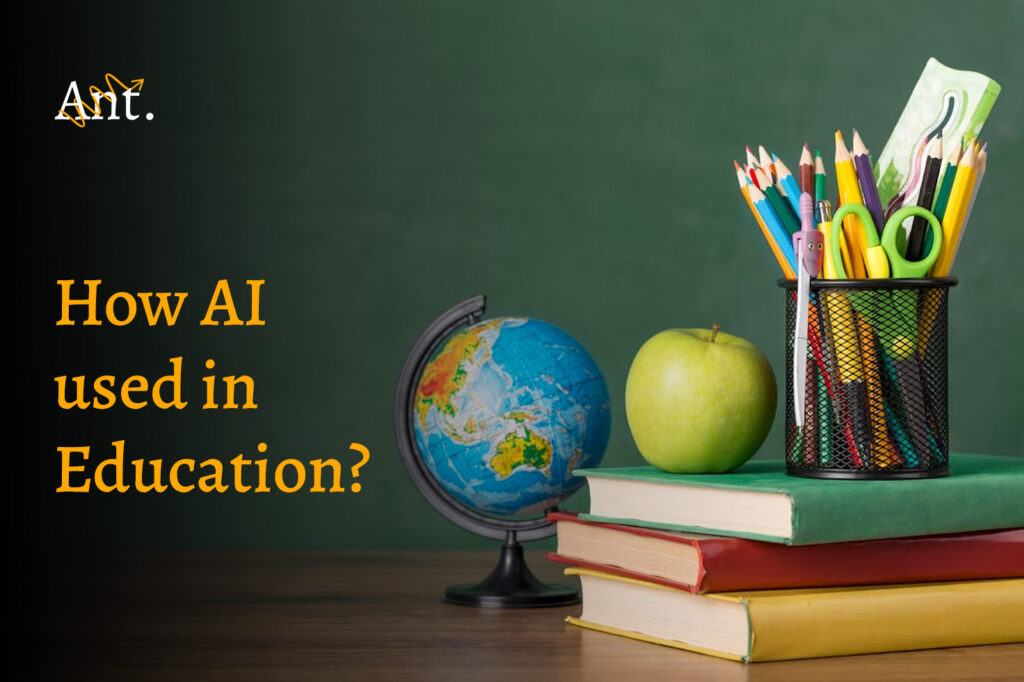
Frequently Asked Questions
1. Can biases in AI algorithms be completely eliminated?
While complete elimination of biases might be challenging, efforts to mitigate biases through data diversification, algorithmic adjustments, and ethical guidelines aim to significantly reduce their impact.
2. How can biases in AI algorithms affect students in educational settings?
Biases can impact students’ learning opportunities, resource allocation, and even career prospects if AI systems make biased recommendations or decisions regarding educational pathways.
3. Do biases in AI algorithms reflect intentional discrimination?
Not necessarily. Biases can emerge unintentionally from historical data or algorithm design, reflecting societal disparities rather than intentional discrimination.
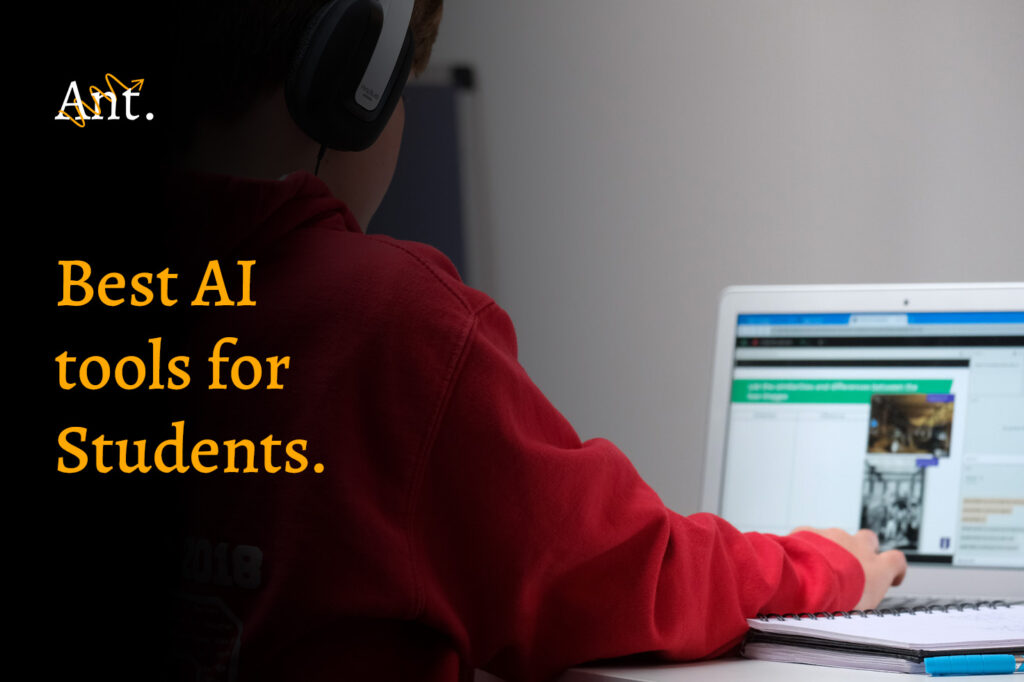
4. How transparent are AI algorithms in terms of their decision-making processes?
Transparency in AI algorithms varies. Efforts are ongoing to develop explainable AI techniques that provide insights into how algorithms arrive at decisions, enhancing transparency and accountability.
5. Are biases in AI algorithms solely related to demographic factors?
No, biases can manifest in various forms beyond demographics, including cultural, linguistic, or socio-economic biases, affecting different aspects of educational experiences.
Conclusion
Addressing biases in AI disadvantages of ai to ensure fair and equitable learning opportunities in education. By understanding the sources of bias and implementing strategies like diverse data collection, continuous monitoring, ethical guidelines, fairness metrics, and collaborative approaches, the education sector can work towards more inclusive AI systems.
While complete eradication of biases may be challenging, a concerted effort to mitigate biases fosters a more equitable learning environment. Educators, developers, policymakers, and stakeholders must collaborate to ensure AI algorithms prioritize fairness and inclusivity, fostering an educational landscape that empowers and benefits all students.